The 16th Edition of the International Conference on Advanced Technologies, Systems, and Services in Telecommunications (TELSIKS’2023) unfolded at the distinguished Faculty of Electronic Engineering (FEE), University of Nis, Serbia, from October 25-26, 2023. This conference marked a significant milestone in the exploration of cutting-edge technologies and services in the field of telecommunications.
The FAAI project consortium was represented by delegations from University of Bielsko-Biala (UBB), University of Library Studies and IT (ULSIT), University of Nis (UNI), University of Ss. Cyril and Methodius in Trnava (UCMT), and University of Montenegro (UoM), collectively showcasing the collaborative efforts and expertise of these esteemed institutions.
Opening Ceremony and Plenary Session: A Glimpse of Our Delegation’s Engagements
Before delving into the special session on Applied Artificial Intelligence, our delegations had the privilege of participating in the distinguished Opening Ceremony and Plenary Session of TELSIKS’2023.
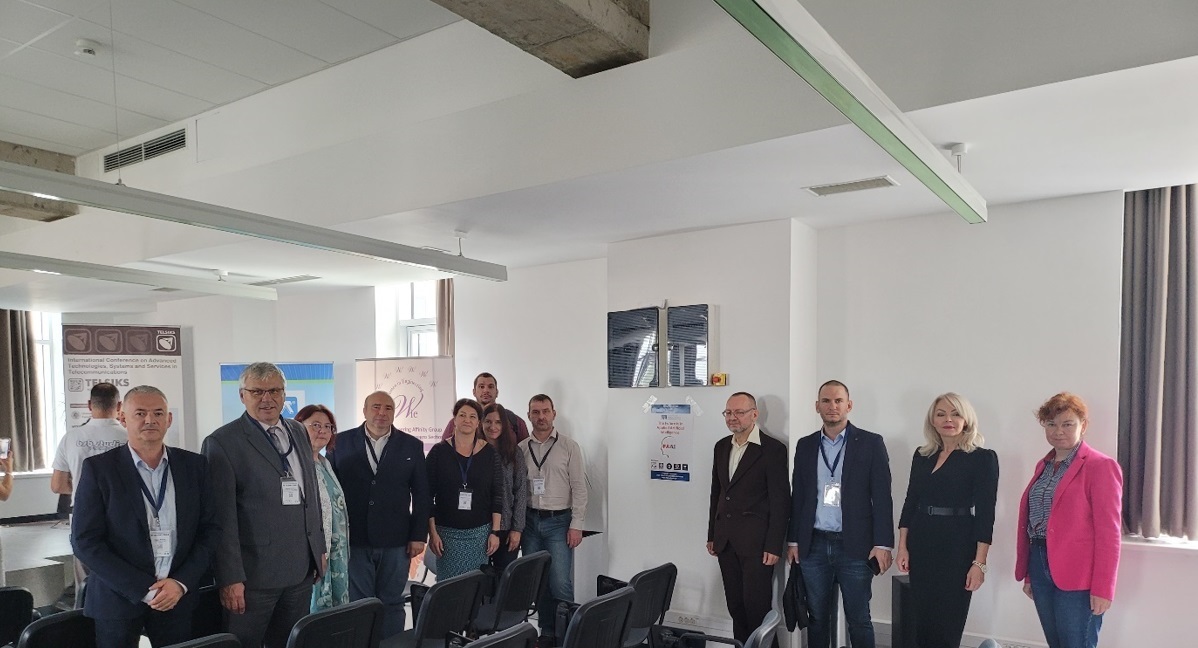
The Opening Ceremony, held in Room 457, FEE, was a momentous occasion marked by an atmosphere of camaraderie and shared purpose. It provided a fitting commencement to the conference, setting the stage for the intellectually stimulating interactions that followed.
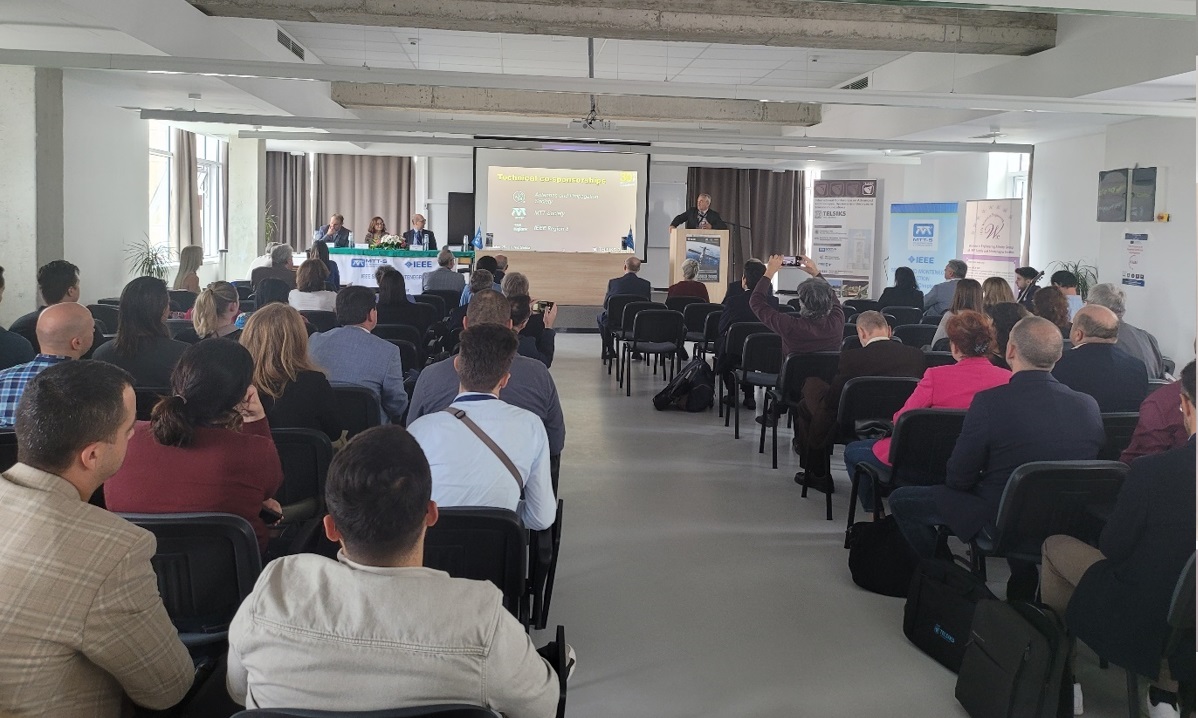
Following this, the Plenary Session, also convened in Room 457, FEE, showcased a lineup of eminent speakers who expounded upon the latest trends and advancements in telecommunications. Our delegations actively engaged in these enriching discussions, contributing to the collective discourse on the future of the field.
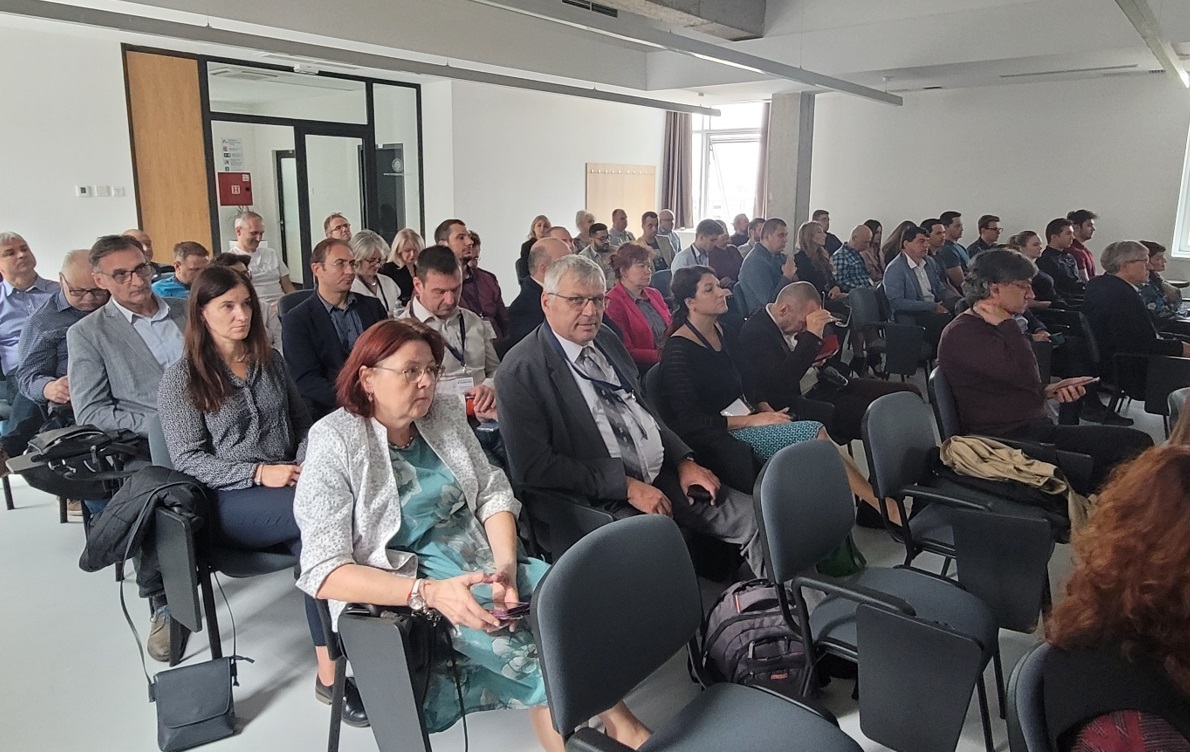
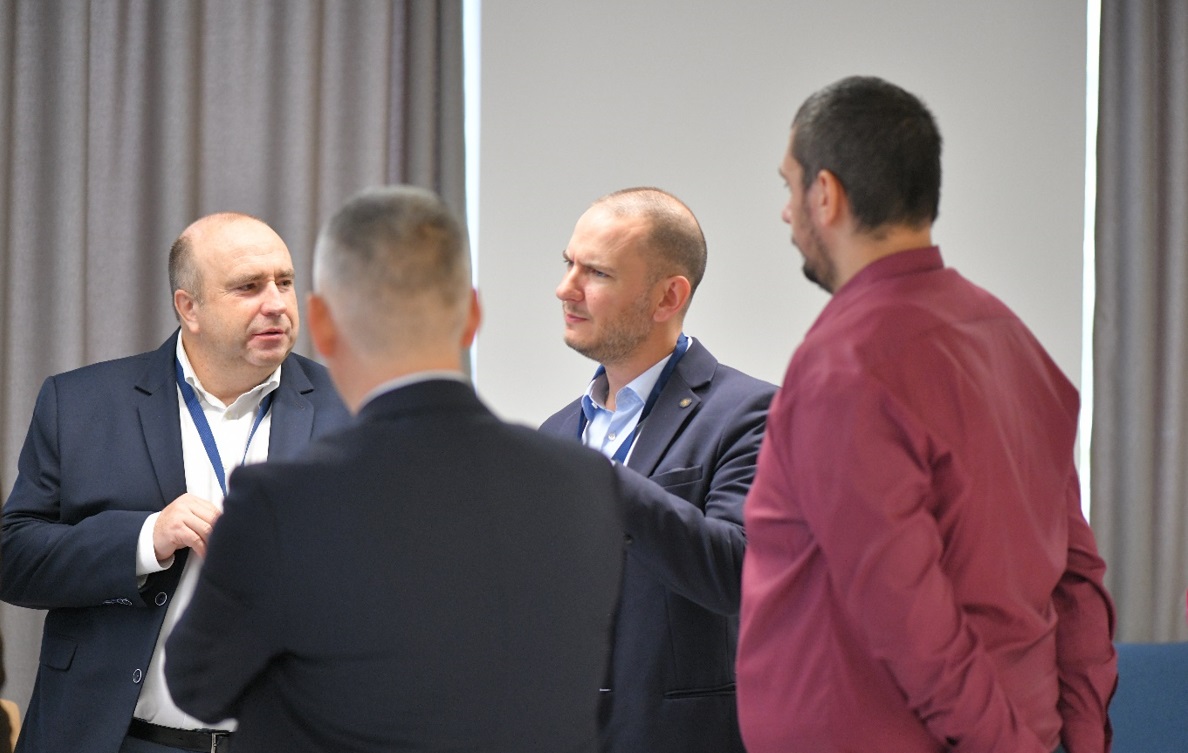
Special Session: Setting up the Applied Artificial Intelligence Learning Requirements (SS3)
A significant highlight of the conference was the special session on Applied Artificial Intelligence (AI) and learning environments. This session delved into the development of a competency framework for Applied AI, encapsulating technical, business, and ethical perspectives, in alignment with the Erasmus+ project FAAI No. 2022-1-PL01-KA220-HED-000088359.
Chaired by Vasyl Martsenyuk and Olivera Pronić-Rancic, the session played a pivotal role in fostering dynamic discussions and facilitating the exchange of innovative ideas on Applied AI.
Noteworthy Talks
1. “On Emerging Methodology for Collection of Good Practices in Applied Artificial Intelligence”
• Vasyl Martsenyuk, George Dimitrov, Dejan Rancic, Iveta Dirgova Luptakova, Igor Jovancevic, Aleksandra Klos-Witkowska, Marcin Bernas, and Tomasz Gancarczyk.
2. “Research and Analysis of IT Specifications of Good Practices in Artificial Intelligence”
• Paulina Tsvetkova, George Dimitrov, Vasyl Martsenyuk, Iveta Dirgová-Luptáková, Dejan Rancic, Igor Jovancevic, Iva Kostadinova, Katia Rasheva-Yordanova, Pepa Petrova, and Pavel Petrov.
3. “Research and Analysis of Different Real Cases, with use of AAI”
• Iva Kostadinova, George Dimitrov, Vasyl Martsenyuk, Dejan Rancic, Iveta Dirgova-Luptakova, Igor Jovancevic, Ivan Trenchev, Stefka Toleva-Stoimenova, and Pavel Petrov.
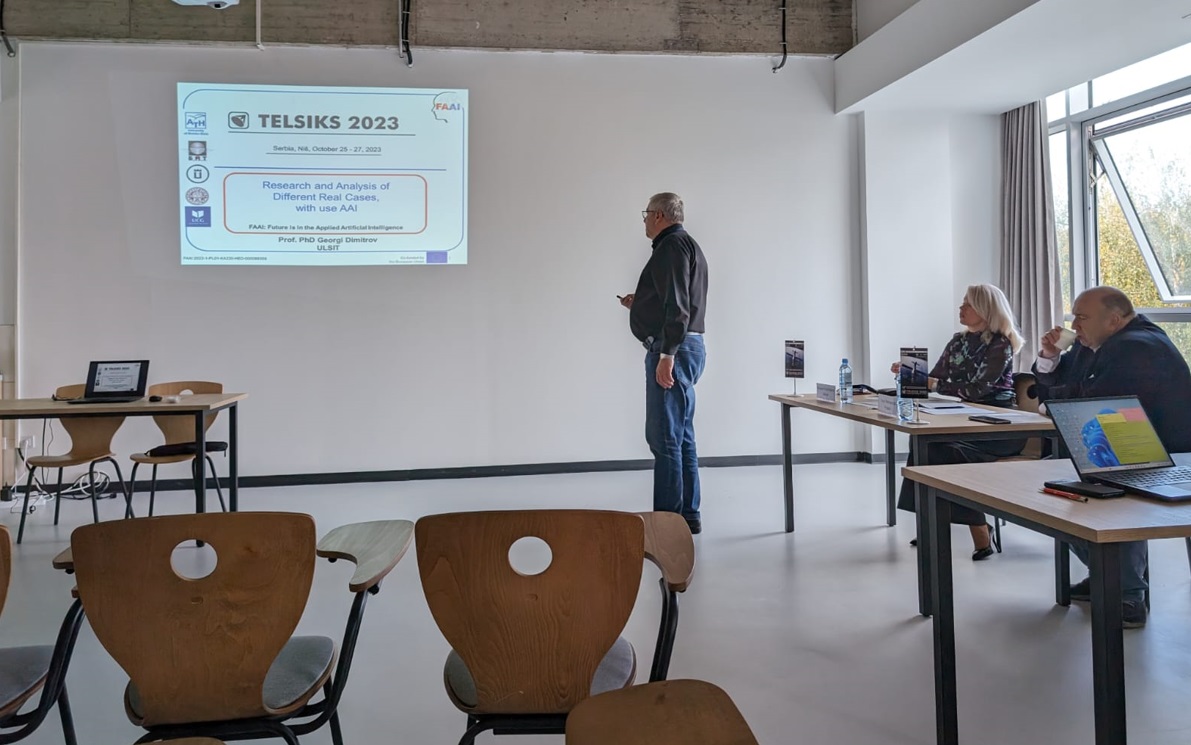
4. “Employer Requirements for Graduate Competencies in Applied Artificial Intelligence”
• Dragan Stojanovic, Olivera Pronić-Rancic, Natalija Stojanovic, Dejan Rancic, and Marko Milojkovic.
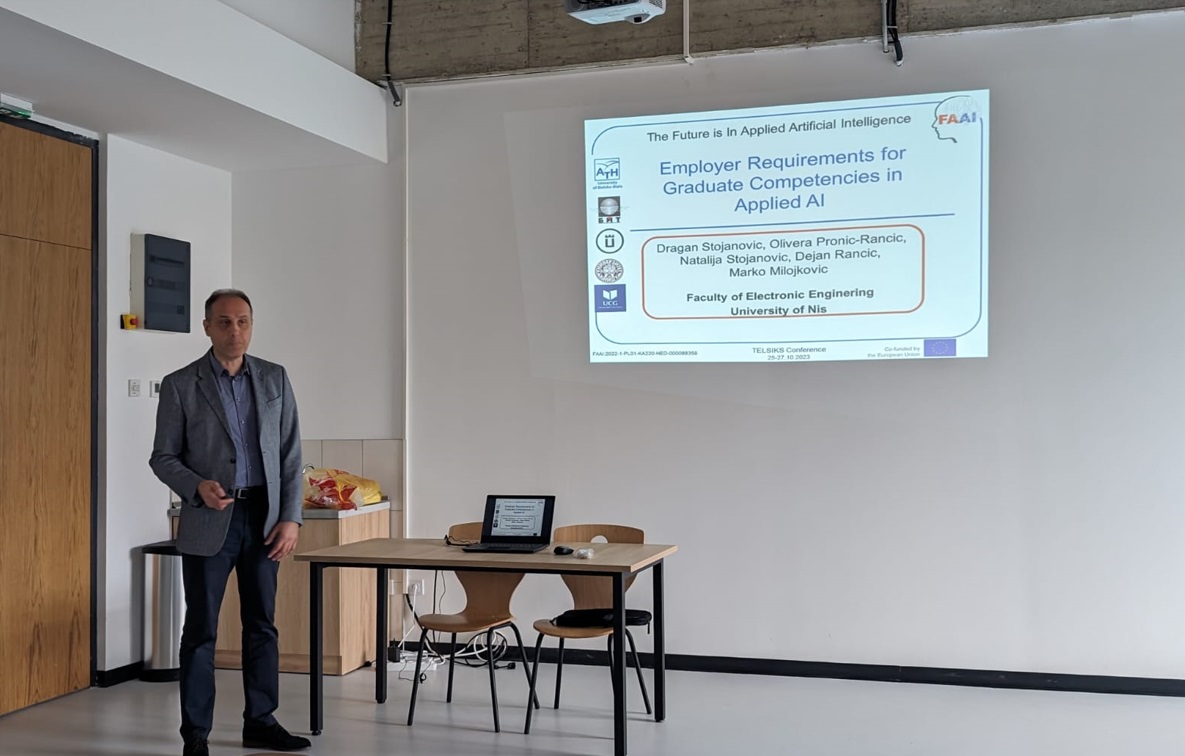
5. “Research and Analysis on the Labor Market in the Field of Applied AI”
• Aleksandar Plamenac, Igor Jovancevic, Georgi Dimitrov, Vasyl Martsenyuk, Dejan Rancic, and Iveta Dirgova-Luptakova.
6. “On Predicting Financial Time Series of Various Granularity as an Applied AI Problem”
• Vasyl Martsenyuk and Jacek Kafel-Kania.
7. “On Manufacturing Network Design as an Applied AI Problem”
• Vasyl Martsenyuk and Nataliia Kit.
Engaging Discourse and Valuable Insights
The ensuing discussions were characterized by a palpable enthusiasm and a profound appreciation for the depth of knowledge shared. The questions posed were astute, and the insights imparted were instrumental in augmenting our collective understanding.
Looking Ahead
The conference culminated as a resounding success, serving as an exemplary platform for the dissemination of the FAAI project’s findings. The strides made in the domain of Applied AI are nothing short of commendable, and we remain sanguine about the transformative potential of our collaborative endeavors.
We extend our gratitude to all participants for their invaluable contributions, and we eagerly anticipate the continued discourse on these vital topics.
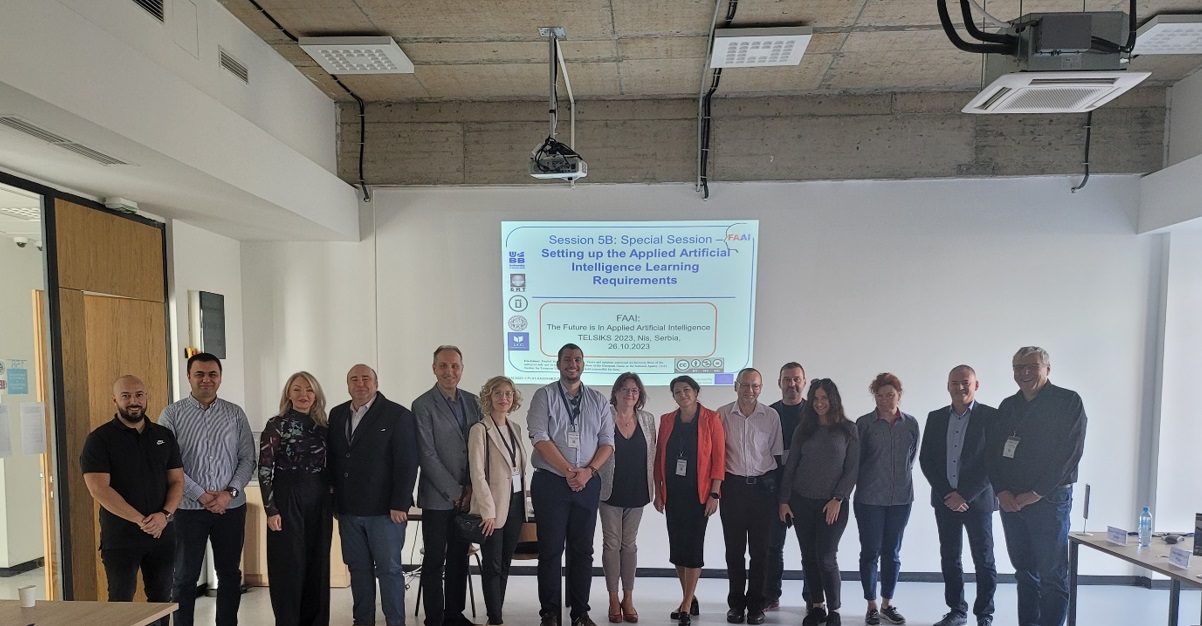